Encog Propogation Training Algorithms
Hello everybody,
today I want to describe in simple words some training algos of Encog.
Before I'll continue, I want to show general block schema of training algorithms:
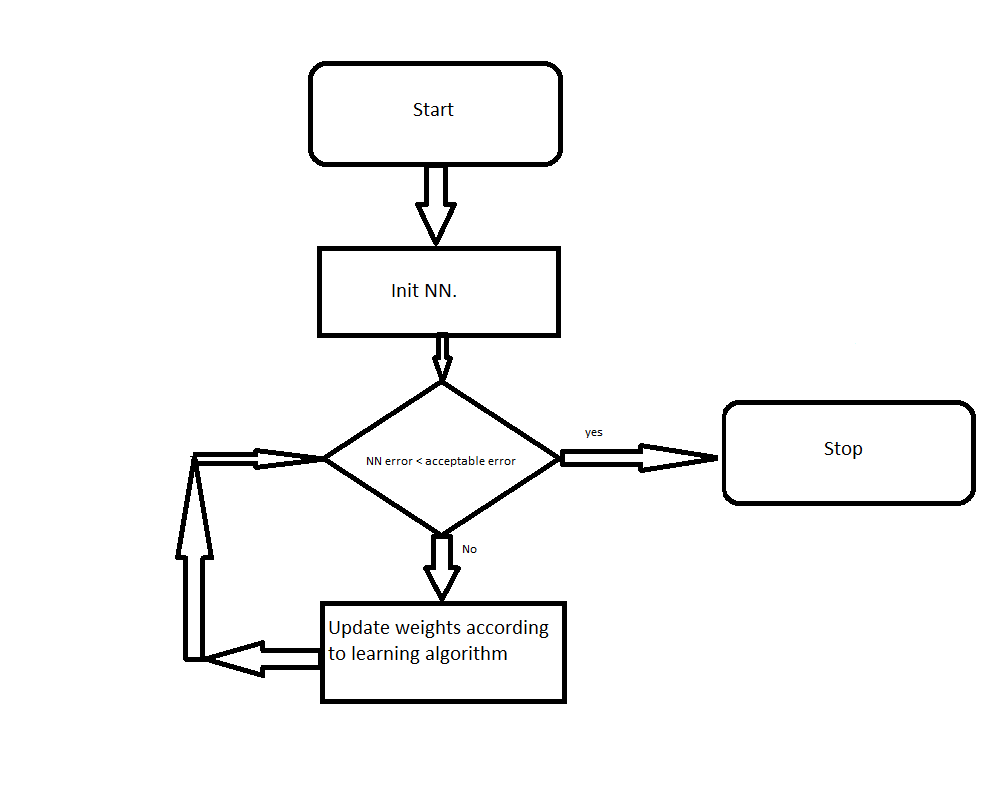
Init NN can look like this:
public BasicNetwork CreateNetwork() { var network = new BasicNetwork(); network.AddLayer(new BasicLayer(WindowSize)); network.AddLayer(new BasicLayer(10)); network.AddLayer(new BasicLayer(1)); network.Structure.FinalizeStructure(); network.Reset(); return network; }
Steps "NN error < acceptable error" -> "Update weights according to learning algorithim" can look like this:
public void Train(BasicNetwork network, IMLDataSet training) { ITrain train = new ResilientPropagation(network, training); int epoch = 1; do{ train.Iteration(); Console.WriteLine(@"Epoch #" + epoch + @" Error:" + train.Error); epoch++; } while (train.Error > acceptableError); }
The first in my decription goes Backpropogation algorithm. Few characteristics of it:
1. probably the oldest training algorithm
2. As name says error are propogated back
3. usage:
var train = new Backpropogation(network, trainingSet, learningRate, momentum);
The next learning algorithm goes Manhattan update rule. Few characteristics of it:
1. use only sign of the gradient
2. Updates weiht by constant value ( depending from the sign it will be added or subtracted from the weithg )
3. usage:
var train = new ManhattanPropogation(network, trainingSet, constantValue);
as usually constantValue is very low ( around 0.00001 )
Other one is Quck propogation algorithm. Features of this method:
1. Newton's method of error minimization
2. Use learning trate which is higher value ( I used values starting from 2 )
3. Usage:
var train = new QuickPropogation(network, trainingSet, learningRate);
Yet one more training algorithm is Resilent propogation algorithm. Some details of this method:
1. One of the fastest algorithm in Encog.
2. Easiest usage ( you don't care about rate, momentum or constant value )
3. It uses sign of gradient value.
4. It tries to compute delta value for each weight and use it for calculated weight.
5. Example of usage:
var train = new ResilentPropogation(network, trainingSet);
6. It has four variants of resilent propogation: RPROP+, RPROP-, iRPROP+, iRPROP- . The best is considered iRPROP+
One more detail goes Scaled conjugate gradient. Features of it:
1. Use conjugate gradient method
2. Not applicable to all dataset
3. Doesn't require any learning paramethers
4. Example of usage:
var train = new ScaledConjugateGradient(network, trainingSet);
And the last one propogation algorithm is Levenberg Marquardt Algorithm ( aka LMA ). This is my favorite method. Features of it:
1. Something in between Gauss-Newton Algorithm ( GNA ) & Gradient Method
2. Easy to use ( no learning parameters )
3. Example of creating:
var train = new LevenbergMarquardtTraining(network, trainingSet);
4. Sometime it can be very efficient.